Credit Risk Analytics
Imagine yourself as a financial institution, entrusted with the weighty responsibility of lending money. Every loan you extend is a calculated leap of faith, a bet on the borrower's future ability to repay. But in the dynamic landscape of finance, where economic tides ebb and flow, how can you confidently assess the risks involved? Enter the fascinating realm of credit risk analytics, a complex tapestry woven from data, models, and expertise. This intricate process equips financial institutions with the foresight to navigate the treacherous waters of lending, ensuring their own stability while fostering economic growth.
Credit risk analytics is, in essence, the art and science of scrutinizing the likelihood that a borrower will default on their loan commitments. It is the meticulous examination of their financial history, current circumstances, and future potential, all weighed against the ever-shifting backdrop of the market. This meticulous process isn't merely a bureaucratic necessity; it is the foundation upon which responsible lending is built, safeguarding the interests of both the lender and the borrower.
"Risk is the price of progress." - John F. Kennedy
To understand the intricacies of credit risk analytics, let's embark on a journey through its core components. We'll navigate each step with a keen eye, exploring the methods employed and the insights gleaned. Think of it as a treasure hunt, where each step brings us closer to understanding the valuable insights hidden within the data.
Table of Contents
Data Collection: Gathering the Clues
The first step in any detective story is gathering evidence, and credit risk analytics is no different. Financial institutions meticulously collect a vast array of data, each piece a clue pointing to the borrower's financial health. This data acts as the foundation upon which all subsequent analyses are built.
Imagine you're a detective investigating a case. You'd start by gathering witness statements, examining crime scene evidence, and reviewing past records. In the world of credit risk, the "crime scene" is the borrower's financial history, and the "evidence" is the data collected from various sources.
Here are some key data sources:
Credit Bureau Data: These reports, compiled by agencies like Experian, Equifax, and TransUnion, offer a snapshot of a borrower's credit history, including their payment history, loan accounts, and credit scores. Think of them as the "official records" of the borrower's financial past.
Financial Statements: These documents provide a detailed view of a borrower's financial position, encompassing their income, expenses, assets, and liabilities. They are akin to the "financial blueprints" of the borrower, revealing their financial strength and weaknesses.
Demographic Data: Age, income, employment status, and other personal characteristics provide context to the borrower's financial situation. They add a human touch to the data, offering a glimpse into the borrower's life circumstances.
Behavioral Data: Purchase behavior, transaction history, and online activity can reveal patterns and trends in a borrower's spending habits. It's like observing the borrower's daily routine, gleaning insights into their financial behavior.
Macroeconomic Data: Economic trends, such as inflation, unemployment rates, and interest rates, provide a broader context for the borrower's financial health. These factors can influence a borrower's ability to repay, highlighting the importance of understanding the economic landscape.
Credit Scoring Models: Deciphering the Patterns
Once the data is collected, the next step is to analyze it. This is where credit scoring models come into play. These models are sophisticated algorithms that use statistical methods to evaluate a borrower's creditworthiness based on their financial history.
Imagine you're a historian studying a series of events. You'd look for patterns and trends to understand the underlying narrative. Credit scoring models do something similar; they analyze the "financial story" of the borrower, seeking patterns that indicate their likelihood of repayment.
The most widely known credit scoring model is the **FICO score**, a numerical representation of a borrower's credit risk. It's a widely used tool in the lending industry, relied upon by banks, lenders, and credit card companies to assess the risk associated with extending credit.
FICO scores are calculated using five key factors:
Payment History: This factor accounts for the borrower's track record of timely payments. It's a testament to their reliability and financial discipline. A history of consistent payments signifies a higher likelihood of repayment.
Amounts Owed: This factor assesses the borrower's current debt levels. A lower amount owed suggests a lower risk of default, as the borrower has more financial breathing room.
Length of Credit History: This factor evaluates the duration of the borrower's credit activity. A longer credit history generally implies a more stable financial history and a lower risk profile.
Credit Mix: This factor considers the diversity of credit accounts the borrower has, such as credit cards, mortgages, and auto loans. A balanced mix suggests a responsible approach to credit management.
New Credit: This factor examines recent credit inquiries and new credit accounts opened. Excessive credit inquiries or new accounts can indicate a need for additional credit or a potential financial strain.
While FICO scores remain a mainstay in the industry, their limitations have spurred the development of more sophisticated models. **Machine learning** algorithms, powered by artificial intelligence, have revolutionized the way credit risk is assessed. These models can analyze vast amounts of data, including alternative data sources, to identify complex patterns and trends that traditional models may miss.
These advanced models can be particularly beneficial when dealing with borrowers who have limited credit history or unconventional financial profiles. They allow for a more nuanced and informed assessment, opening the door to credit access for individuals who might otherwise be overlooked by traditional methods.
Probability of Default: Predicting the Unpredictable
Once a borrower's creditworthiness has been assessed, the next step is to estimate the likelihood of default. This is where the concept of **Probability of Default (PD)** comes into play. It's a key element in credit risk analytics, providing a quantitative measure of the borrower's potential risk.
Imagine you're a weather forecaster predicting the likelihood of rain. You'd use historical data, current conditions, and scientific models to make your predictions. In credit risk analytics, PD is similar to the weather forecast, providing a probabilistic assessment of the borrower's likelihood of defaulting on their loan.
PD is typically calculated using statistical models, including:
Logistic Regression: This statistical technique uses a mathematical equation to predict the probability of a binary outcome (in this case, default or no default) based on a set of independent variables, such as credit scores, debt levels, and income.
Decision Trees: These models use a series of branching decisions based on specific criteria to predict the likelihood of default. It's like following a "decision tree" to reach a conclusion about the borrower's risk.
Random Forests: This ensemble learning method uses multiple decision trees to make predictions. It's like having a team of experts, each with their own "decision tree," working together to arrive at a more accurate assessment of the borrower's risk.
Neural Networks: These models are inspired by the human brain, using interconnected nodes to learn from data and make predictions. They can handle complex relationships and patterns in the data, making them particularly effective in analyzing large datasets.
In recent years, machine learning algorithms such as Gradient Boosting and XGBoost have emerged as powerful tools for calculating PD. These algorithms are known for their ability to handle vast datasets, identify complex relationships, and improve predictive accuracy. They are increasingly used in credit risk analytics to refine models and enhance their predictive power.
Loss Given Default: Mitigating the Losses
Even with advanced models and meticulous analysis, defaults can still occur. The crucial next step is to assess the potential financial impact of such defaults. This is where the concept of Loss Given Default (LGD) comes into play. LGD is a measure of the percentage of the total loan amount that is lost when a borrower defaults, taking into account any recovery through collateral or other means.
Imagine you're a farmer facing a drought. You'd want to estimate the potential loss of crops and take steps to mitigate the damage. In credit risk analytics, LGD is like the "drought assessment" for lenders, helping them understand the potential financial loss associated with a default and plan accordingly.
LGD is typically calculated using two main methods:
Historical Loss Data Analysis: This method relies on historical data of past loan defaults to estimate the average percentage of losses experienced. It's like looking back at previous droughts to understand the typical crop losses experienced.
Regression Models: These models use borrower characteristics and loan specifics to predict the potential loss associated with a default. This approach is like using meteorological models to predict the severity of a future drought based on current conditions and historical patterns.
Understanding LGD is crucial for developing risk mitigation strategies. If a lender anticipates a high LGD for a particular loan, they may require more collateral, adjust loan terms, or even decline the loan altogether. This proactive approach helps minimize financial losses in the event of a default.
Exposure at Default: Quantifying the Stakes
The next step in the credit risk analytics journey is to determine the amount of money the lender is exposed to at the time of default. This is known as the Exposure at Default (EAD). It represents the total amount of money the lender would potentially lose if the borrower defaults on their loan.
Imagine you're a casino owner. You'd want to know the maximum amount of money a gambler could potentially win, helping you manage your risk. In credit risk analytics, EAD is like the casino's "exposure limit," representing the maximum potential loss from a loan.
EAD typically considers the total loan amount but can also include accrued interest or other associated costs. For loans with dynamic credit limits, such as credit cards, analytical models are used to forecast future exposures based on the borrower's spending behavior and credit limits.
Understanding EAD is critical for financial institutions to effectively manage their risk exposure. It allows them to assess the potential financial impact of a default and allocate capital accordingly. By monitoring EAD and adjusting loan terms or credit limits as needed, institutions can proactively minimize their exposure to potential losses.
Expected Loss: A Unified Risk Metric
Having calculated PD, LGD, and EAD, we now have a comprehensive picture of the potential financial impact of a default. The final step is to combine these three components into a single risk measure known as the Expected Loss (EL). EL represents the expected average loss the lender is likely to incur on a loan over its lifetime.
Imagine you're a financial advisor assessing the risk of investing in a particular stock. You'd consider the potential for gains, losses, and the probability of each outcome. EL is similar to the "expected return" of an investment, providing a unified measure of the potential financial risk associated with a loan.
The formula for calculating EL is straightforward:
Expected Loss (EL) = PD x LGD x EAD
EL is a powerful tool for financial institutions to make informed decisions about loan approvals, pricing, risk mitigation strategies, and capital allocation. By understanding the expected loss for a given loan or loan portfolio, institutions can better manage their risk profile, allocate capital efficiently, and ensure their financial stability.
Stress Testing: Conquering the Storms
Credit risk analytics is not static; it must constantly adapt to changing market conditions. This is where **stress testing** comes into play. Stress testing involves simulating adverse economic conditions to evaluate the resilience of the loan portfolio under extreme scenarios.
Imagine you're an architect designing a building in an earthquake-prone region. You'd use simulations to test the building's structural integrity under seismic stress. In credit risk analytics, stress testing is like the "earthquake simulation" for lenders, testing the robustness of their loan portfolios under challenging conditions.
Common stress test scenarios include:
Economic Downturns: This scenario simulates a recession, with increased unemployment rates, lower consumer spending, and reduced business activity, potentially impacting the borrower's ability to repay.
Rising Unemployment Rates: This scenario models a rise in unemployment, potentially affecting borrowers' job security and their capacity to repay loans.
Interest Rate Hikes: This scenario simulates an increase in interest rates, which can make it more expensive for borrowers to repay their loans, potentially increasing the risk of default.
Industry-Specific Challenges: These scenarios assess the impact of specific industry challenges, such as technological disruptions, regulatory changes, or market volatility, on the borrower's business and their ability to repay loans.
Stress testing helps institutions understand the potential impact of adverse conditions on their loan portfolios. It allows them to identify vulnerabilities, develop contingency plans, and strengthen their risk mitigation strategies. This proactive approach ensures they are better prepared to weather economic storms and maintain financial stability.
Credit Portfolio Management: Optimizing the Whole
While credit risk analytics is crucial for assessing individual loans, financial institutions must also consider the overall risk of their entire loan portfolio. This is where **credit portfolio management** comes into play. It focuses on optimizing the mix of credit exposures to minimize overall risk and maximize returns.
Imagine you're an investor building a diversified portfolio of stocks and bonds. You'd consider different asset classes, risk profiles, and potential returns to create a balanced portfolio. Credit portfolio management is similar, aiming to diversify and optimize the lender's overall risk exposure across different types of loans and borrowers.
Key techniques used in credit portfolio management include:
Risk Diversification: Spreading risk across different industries, geographies, or types of loans. This approach is similar to diversifying an investment portfolio, reducing the overall risk by minimizing concentration in any single sector or asset class.
Risk-Adjusted Return on Capital (RAROC): Calculating expected returns while adjusting for the risk involved. RAROC helps institutions prioritize investments that offer the highest potential returns while balancing risk considerations. It's like evaluating investment opportunities based on their potential reward and risk.
Portfolio Stress Testing: Evaluating the impact of macroeconomic shifts on the entire portfolio. This approach is similar to stress testing individual loans but at the portfolio level, allowing institutions to assess the overall risk and resilience of their loan portfolio under adverse conditions.
Credit portfolio management is essential for financial institutions to achieve a sustainable balance between risk and reward. By strategically managing their loan portfolios, institutions can optimize returns, minimize overall risk, and ensure their long-term financial stability.
Regulatory Requirements: Navigating the Legal Landscape
Credit risk analytics is not just about data and models; it is also subject to a complex web of regulations designed to protect financial institutions, consumers, and the overall economy. Financial institutions must adhere to these regulations, ensuring their risk management practices are compliant and transparent.
Imagine you're a company operating in a regulated industry. You must comply with specific laws, guidelines, and reporting requirements. Credit risk analytics is similar, operating within a legal framework that shapes the way institutions assess and manage risk.
One of the most prominent regulatory frameworks for credit risk is the Basel Accords, a set of international standards for banking supervision. The Basel Accords (Basel II and Basel III) require financial institutions to maintain adequate capital based on their risk exposures. These regulations have a significant impact on the way institutions model risk, calculate capital reserves, and manage their loan portfolios.
Compliance with regulatory requirements is essential for financial institutions to operate within the legal framework and maintain their reputation. It ensures their activities are transparent, responsible, and contribute to the stability of the financial system.
Machine Learning and AI in Credit Risk: The Future is Now
The advent of machine learning and artificial intelligence (AI) has revolutionized the landscape of credit risk analytics. These technologies offer unprecedented power to analyze vast amounts of data, identify complex patterns, and predict outcomes with greater accuracy.
Imagine you're a doctor using advanced diagnostic tools to detect diseases earlier and more accurately. Machine learning and AI are like the "advanced diagnostic tools" in credit risk analytics, allowing for more sophisticated and insightful risk assessments.
Here are some key applications of machine learning and AI in credit risk:
Predictive Models: Machine learning models can analyze complex datasets, including alternative data sources, to more accurately predict defaults. This can lead to better loan approvals, more tailored pricing, and improved risk mitigation strategies.
Alternative Data Sources: AI can integrate non-traditional data sources, such as social media activity, online behavior, and mobile phone usage, to assess creditworthiness. This can broaden the scope of analysis and provide a more comprehensive picture of the borrower's financial health.
Real-Time Risk Assessment: AI-driven systems can continuously monitor changing conditions and borrower behavior to provide updated risk assessments. This allows for more dynamic and responsive risk management, adapting to evolving circumstances in real time.
The use of machine learning and AI in credit risk analytics is still in its early stages, but its potential is immense. These technologies have the power to transform the way credit risk is assessed, managed, and mitigated, leading to more efficient, transparent, and inclusive financial systems.
Challenges: The Unforeseen Obstacles
Despite the incredible advances in credit risk analytics, there are still challenges to overcome. These challenges require careful consideration and innovative solutions to ensure the accuracy, fairness, and effectiveness of risk assessments.
Imagine you're a scientist conducting an experiment. You must carefully control variables, ensure accuracy, and address potential biases to ensure the validity of your results. In credit risk analytics, these same principles apply, ensuring the robustness and reliability of risk assessments.
Here are some key challenges:
Data Quality: Inaccurate or incomplete data can lead to flawed risk assessments. It's like building a house on a shaky foundation; the structure will be unstable and prone to collapse. Ensuring data quality is crucial for accurate and reliable risk assessments.
Model Validation: Ensuring that models perform accurately over time and in changing market conditions. It's like regularly calibrating your compass to ensure it's pointing in the right direction. Regularly validating models helps maintain their accuracy and relevance in a dynamic environment.
Bias in Models: Traditional credit models may sometimes unintentionally favor or discriminate against certain groups. This can lead to unfair outcomes and perpetuate existing inequalities. Machine learning models, if not carefully designed, can also inherit bias from the data used to train them. It's important to be vigilant about bias and implement mechanisms to mitigate its impact.
Regulatory Compliance: Financial institutions must ensure their models and strategies are compliant with industry regulations and governance standards. It's like navigating a complex maze of laws and regulations; adhering to these rules ensures that institutions operate within legal boundaries and maintain their credibility.
Conclusion: Building a Sustainable Future
Credit risk analytics is a multifaceted and constantly evolving field, driven by data, technology, and a commitment to responsible lending. By embracing innovation, addressing challenges, and fostering a culture of transparency and ethical decision-making, financial institutions can build a more sustainable and equitable future for themselves and their borrowers.
As we stand at the intersection of data, models, and human judgment, the future of credit risk analytics holds immense potential. By harnessing the power of technology while remaining mindful of ethical considerations, we can navigate the labyrinth of lending, creating a financial landscape that is both responsible and conducive to growth.
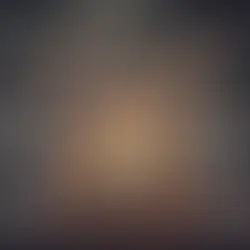
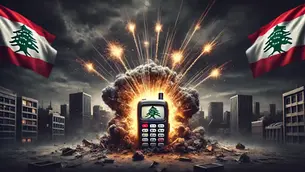
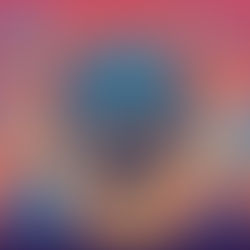
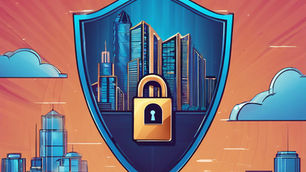
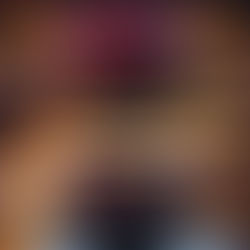
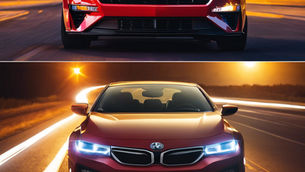
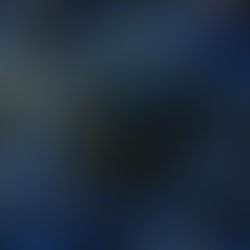
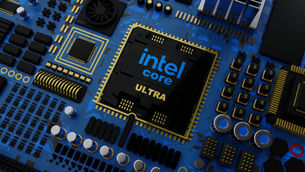