Credit Risk Modelling
Imagine a world where lenders could predict with absolute certainty whether a borrower would repay their loan. Sounds like a utopia, doesn't it? While perfect foresight remains elusive, the realm of credit risk modeling offers a powerful lens to decipher the complex tapestry of borrower behaviour and financial health. This field, a cornerstone of financial institutions, plays a pivotal role in driving lending decisions, crafting interest rates, and ultimately, safeguarding the financial system. It's a fascinating blend of statistics, data science, and a dash of human intuition.
As we embark on this journey into the depths of credit risk modeling, let's begin by acknowledging that the very act of lending inherently carries risk. It's a gamble, a calculated risk, where lenders hope for the best but prepare for the worst. Credit risk modeling, therefore, is not about eliminating risk altogether, but rather, about quantifying and managing it. It's about bringing order to the chaos, transforming the nebulous fear of default into a tangible, measurable probability.
"The best way to predict the future is to create it." - Abraham Lincoln
Unveiling the Layers of Credit Risk
Credit risk, in its purest form, is the chance that a borrower will fail to meet their financial obligations, leaving the lender out of pocket. It's a multifaceted beast, encompassing various types of risk.
1. Default Risk: The Shadow of Non-Payment
Default risk is the most basic form of credit risk, representing the possibility that a borrower will simply not repay their loan as agreed. It's the ominous shadow that looms over every loan agreement, reminding lenders that even the most promising borrower can stumble. Imagine, for instance, a young entrepreneur taking out a loan to launch a new business. While their vision may be compelling, unforeseen market conditions or operational challenges could derail their plans, leading to default.
2. Exposure Risk: The Potential Depth of Loss
Exposure risk goes beyond the mere possibility of default, quantifying the potential amount of money a lender could lose in case of a default. It's like a scale, weighing the lender's exposure to the borrower's financial health. Take a bank loaning a significant amount to a large corporation. If the company defaults, the bank's exposure, that is, the amount they could lose, would be substantial.
3. Recovery Risk: The Hope of Recouping Losses
Recovery risk is the elusive glimmer of hope in the face of default. It's the likelihood that a lender can recover some of their funds even if a borrower defaults. Imagine a borrower defaulting on a secured loan, where the lender has collateral, such as a property. If the lender can successfully sell the collateral, they may be able to recover some of their losses.
The Building Blocks of Credit Risk Models
To tame the wild beast of credit risk, financial institutions rely on sophisticated models, often powered by data science and machine learning techniques. These models are built on a foundation of key components.
1. Probability of Default (PD): The Likelihood of Failure
The Probability of Default (PD) is the heart of any credit risk model, representing the likelihood that a borrower will default on their loan within a specific timeframe. It's like a weather forecast for financial health, attempting to predict the chance of a "storm" - a default - occurring. Banks employ various statistical methods and data analysis to calculate PD, taking into account factors like the borrower's credit history, financial statements, and even macroeconomic conditions.
2. Loss Given Default (LGD): The Depth of the Wound
Loss Given Default (LGD) is the grim reality of default, measuring the proportion of the lender's exposure that will be lost if the borrower fails to repay. It's like assessing the damage caused by a financial storm. Factors like collateral availability, the borrower's ability to repay after default, and the potential for recovery play a role in determining LGD. Imagine, for instance, a bank lending money to a borrower who owns a valuable property. If the borrower defaults, the bank can seize the property and sell it to offset some of their losses, lowering the LGD.
3. Exposure at Default (EAD): The Magnitude of the Exposure
Exposure at Default (EAD) is the amount of money a lender is exposed to at the time of default. It's like the total area a storm covers, representing the total amount of money that could be lost. EAD includes not only the principal loan amount but also any accrued interest and other expenses associated with the loan.
4. Expected Loss (EL): The Sum of All Fears
Expected Loss (EL) is the culmination of the previous three components. It's the estimated amount of money a lender expects to lose on a loan due to default. It's the calculated risk, the "expected storm damage," and it's calculated using the following formula:
The Expected Loss (EL) provides a crucial piece of information for financial institutions, helping them to allocate capital reserves and manage their portfolio risk.
5. Stress Testing: The Resilience Test
Stress testing is the crucible in which credit risk models are tempered. It involves evaluating how a portfolio of loans would perform under extreme economic conditions, such as a financial crisis or a sudden spike in interest rates. It's like simulating a "hurricane" to see how the financial infrastructure holds up. By exposing models to these hypothetical stress scenarios, financial institutions can gauge their resilience and identify potential vulnerabilities.
Navigating the Approaches to Credit Risk Modeling
The realm of credit risk modeling is a rich tapestry of approaches, each with its strengths and weaknesses. Let's explore the different avenues that financial institutions can take.
1. Traditional Approaches: The Tried and True
Traditional approaches to credit risk modeling have been honed over decades, often relying on statistical methods that have stood the test of time.
a. Logistic Regression: Predicting Default Probabilities
Logistic regression, a cornerstone of statistical modeling, is widely used in credit scoring. It's a powerful tool for estimating the probability of default based on various factors like income, credit history, and debt levels. The model identifies the relationship between these factors and the probability of default, allowing lenders to assess the risk profile of borrowers. Imagine a bank using logistic regression to analyze loan applications, scoring each applicant based on their likelihood of defaulting.
b. Discriminant Analysis: Separating the Wheat from the Chaff
Discriminant analysis separates borrowers into two distinct groups: defaulters and non-defaulters. It does this by analyzing financial ratios, credit scores, and other relevant factors to distinguish between the two groups. It's like sorting borrowers into two separate baskets, one for those who are more likely to default, and one for those who are less likely.
c. Altman Z-Score: Predicting Bankruptcy
The Altman Z-Score, developed by Edward Altman, is a formula used to predict the likelihood of a company going bankrupt. It considers five key financial ratios, providing a "health score" for the company. The higher the score, the less likely the company is to go bankrupt. Imagine a financial analyst using the Z-Score to assess the financial health of a company before making an investment decision.
2. Modern Approaches: Embracing the Power of Data
With the advent of machine learning and artificial intelligence, credit risk modeling has taken a quantum leap forward. These modern approaches leverage the power of data, enabling financial institutions to develop more sophisticated and accurate models.
a. Machine Learning Models: Unlocking the Secrets of Data
Machine learning models, such as decision trees, random forests, gradient boosting machines (GBM), and neural networks, are revolutionizing credit risk modeling. These models can analyze vast amounts of data, uncovering complex patterns and relationships that might escape human scrutiny. It's like giving a computer a magnifying glass to examine the intricate details of borrower behaviour and financial trends.
b. Support Vector Machines (SVM): Finding the Dividing Line
Support Vector Machines (SVM) are a powerful classification tool, finding the best way to separate borrowers into two groups - those who are more likely to default and those who are less likely. SVM creates a "hyperplane" that divides the data, enabling lenders to make informed decisions about loan approvals.
c. Artificial Neural Networks (ANN): The Simulating Brain
Artificial Neural Networks (ANN) are sophisticated models inspired by the structure of the human brain. They learn from data by adjusting their internal "neurons," creating complex relationships between different factors. It's like giving a computer a brain capable of mimicking the human thought process, enabling it to understand the nuances of credit risk. ANNs are particularly adept at handling complex data patterns and making accurate predictions, making them ideal for credit risk modeling. Imagine a bank using an ANN to analyze a borrower's transaction history, spending patterns, and social media interactions to assess their creditworthiness.
Fueling the Engine: Data at the Heart of Credit Risk Modeling
Just like a car needs fuel to run, credit risk models require data to operate. Data fuels the engine of these models, providing the raw material for predicting default probabilities and managing risk.
1. Credit History: A Window into the Past
Credit history is the fundamental building block of credit risk modeling. It provides a comprehensive record of a borrower's past financial behaviour, including loan repayment history, credit score, and any past defaults. It's like a historical record, revealing the borrower's past financial decisions and their ability to manage debt.
2. Financial Statements: A Snapshot of the Present
Financial statements provide a detailed snapshot of a borrower's current financial health. They reveal information about revenue, expenses, assets, and liabilities, offering a clear picture of the borrower's financial standing. It's like a financial "selfie," showing the borrower's current financial situation.
3. Macroeconomic Data: The Global Context
Macroeconomic data, such as GDP growth, interest rates, and unemployment rates, provides a broader context for understanding the borrower's financial situation. It's like the big picture, revealing the economic landscape that influences the borrower's ability to repay their loan.
4. Behavioral Data: The Nuances of Behaviour
Behavioral data, including transaction data, spending habits, and interactions with the bank, offers valuable insights into the borrower's financial behaviour. It's like a diary, recording the borrower's daily financial activities, providing a more nuanced understanding of their financial habits.
Navigating the Regulatory Labyrinth: Shaping the Landscape of Credit Risk Modeling
The world of credit risk modeling is not a free-for-all. It operates within a complex framework of regulations, designed to ensure financial stability and protect consumers. These regulations set the stage for the development and use of credit risk models.
1. Basel Accords: The Pillars of Global Banking Regulation
The Basel Accords, a series of international banking regulations, play a crucial role in shaping the landscape of credit risk modeling. These accords, designed to strengthen the global financial system, set capital requirements for banks, including provisions for credit risk. Basel II introduced the internal ratings-based (IRB) approach, allowing banks to develop their own models for assessing credit risk. However, Basel III imposed more stringent capital requirements, pushing banks to refine their models further. Imagine the Basel Accords as a set of building codes for the financial system, ensuring that banks are adequately equipped to handle credit risk.
2. IFRS 9: Accounting for Expected Credit Losses
IFRS 9, an accounting standard, requires financial institutions to account for expected credit losses (ECL) when determining provisions for loan defaults. This means that banks must estimate potential losses based on the likelihood of default and the amount they could lose. It's like an accounting system that anticipates future financial storms, requiring banks to build reserves accordingly. This approach, unlike the traditional incurred loss model, takes a more proactive approach to risk management, ensuring that banks are better prepared to handle potential defaults.
Building a Credit Risk Model: A Step-by-Step Guide
Developing a credit risk model is a meticulous process, akin to building a house. Each step is crucial, ensuring that the final model is robust, accurate, and reliable.
1. Data Collection and Preprocessing: The Foundation
The journey begins with data collection, gathering financial, behavioral, and demographic data about borrowers. This is like laying the foundation for the model, providing the building blocks for analysis. The data must be carefully cleaned and prepared to ensure accuracy and consistency, like removing debris and leveling the ground for a solid foundation.
2. Feature Engineering: Selecting the Key Variables
Feature engineering is the art of selecting and creating relevant variables (features) that will help predict credit risk. It's like choosing the right building materials for the house, ensuring that they are strong and suitable for the intended purpose. Feature engineering involves identifying variables that have a significant impact on default probability, such as income, credit score, and debt-to-income ratio.
3. Model Building: Putting the Pieces Together
Model building is the core of the process, using statistical or machine learning techniques to develop a model that predicts default probability or expected loss. It's like assembling the house, putting the different pieces together to create a functional structure. This step involves selecting the appropriate model, training it on the available data, and evaluating its performance.
4. Model Validation: Testing the Strength of the Structure
Model validation is the process of assessing the model's performance using various metrics like accuracy, precision, recall, and ROC/AUC. It's like testing the strength and stability of the house before it is occupied. This step involves comparing the model's predictions with actual outcomes, ensuring that it accurately reflects real-world scenarios.
5. Stress Testing and Scenario Analysis: Simulating the Storms
Stress testing and scenario analysis involve testing the model under various economic conditions, including financial crises and sudden changes in interest rates. It's like simulating storms to see how the house withstands extreme weather. This step ensures that the model is resilient to unexpected events and can accurately predict outcomes under different market conditions.
6. Model Deployment: Bringing the House to Life
Model deployment is the process of integrating the model into the decision-making process, such as loan approval systems. It's like moving into the house and starting to use it. This step involves making the model operational and ensuring that it is seamlessly integrated with the existing processes.
7. Ongoing Monitoring: Ensuring the House Remains Strong
Ongoing monitoring involves continuously validating and recalibrating the model to ensure it adapts to new data and changing market conditions. It's like regularly maintaining the house, ensuring that it remains safe and functional. This step is essential for ensuring that the model remains accurate and relevant over time.
Credit Scoring Models: Measuring the Risk
Credit scoring models provide a numerical assessment of a borrower's creditworthiness, enabling lenders to quickly and efficiently assess risk. They are a crucial tool for managing credit risk.
1. FICO Score: The Industry Standard in the United States
The FICO Score, developed by Fair Isaac Corporation, is the most widely-used credit score in the United States. It is based on consumer credit history, including payment history, amounts owed, length of credit history, new credit, and credit mix. It's like a standardized test for creditworthiness, providing a comprehensive assessment of a borrower's financial history.
2. VantageScore: The Alternative Credit Score
VantageScore, an alternative credit score, was developed by the three major credit bureaus. It is designed to be more inclusive than the FICO Score, taking into account a wider range of credit data, including non-traditional sources like rental payments and utility bills. It's like a broader assessment, looking beyond the traditional credit history to capture a more complete picture of the borrower's financial behaviour.
3. Z-Score (Altman): Predicting Corporate Bankruptcy
The Altman Z-Score, a formula used to predict the likelihood of a company going bankrupt, is frequently employed in corporate credit risk modeling. It is used to assess the financial health of corporations, helping investors and lenders to make informed decisions.
Challenges in the Realm of Credit Risk Modeling
The journey of credit risk modeling is not without its obstacles. Navigating this complex terrain requires a deep understanding of the challenges that lie ahead.
1. Data Quality: The Foundation of Accuracy
Poor data quality, missing data, or outliers can lead to inaccurate predictions. It's like building a house on shaky ground, where the foundation is weak and prone to collapse.
2. Model Bias: Ensuring Fairness and Equity
Machine learning models can be biased if not carefully designed, leading to unfair treatment of certain demographic groups. It's like creating a house with a bias towards a particular type of resident, excluding others from enjoying its benefits.
3. Macroeconomic Uncertainty: The Unpredictable Storms
Economic downturns or unexpected events like the COVID-19 pandemic can cause traditional models to perform poorly. It's like encountering a sudden and unexpected storm that the house is not designed to withstand.
4. Regulatory Compliance: Keeping Up with the Rules
Constant changes in regulations require institutions to frequently update their models. It's like adapting the house to changing building codes and regulations to maintain its safety and compliance.
Conclusion: The Future of Credit Risk Modeling
Credit risk modeling is an essential tool for financial institutions to navigate the ever-changing landscape of risk and ensure profitability. It's a powerful tool for understanding borrower behaviour, predicting potential defaults, and making informed lending decisions. As technology evolves, we can expect to see even more sophisticated and accurate credit risk models, leveraging the power of artificial intelligence and machine learning to unlock new insights and enhance risk management strategies. The journey of credit risk modeling is a dynamic one, constantly evolving with new technologies, changing regulations, and a growing understanding of human behaviour. It's a field that requires constant innovation and adaptation, embracing the challenge of harnessing data to make better decisions in a world of uncertainty.
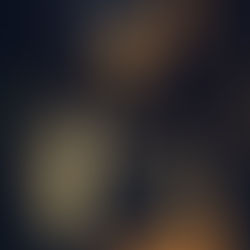

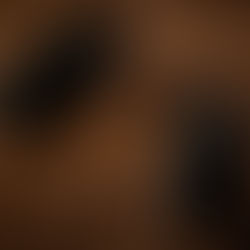
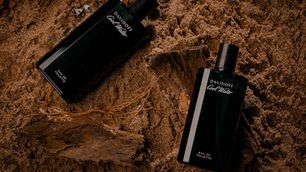
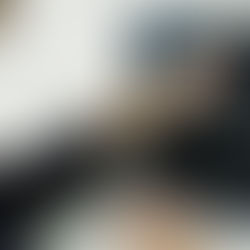
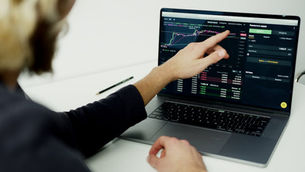

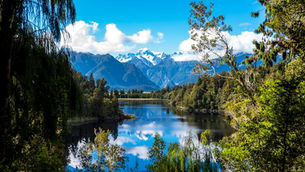