CREDIT SCORING
Imagine a world where your access to financial resources, from a simple loan to a mortgage, hinges on an intricate system of algorithms. This is the reality we live in, a world where credit scoring models silently orchestrate our financial destinies. These statistical tools, wielded by lenders and financial institutions, analyze a vast array of data to determine your creditworthiness, influencing everything from interest rates to the very possibility of getting approved for a loan.
Credit scoring, in essence, is a meticulous dance of data, where algorithms meticulously evaluate your financial history and current behavior. It's like a complex puzzle, where every piece, every transaction, every payment, contributes to forming a holistic picture of your financial reliability. While this might seem daunting, understanding the mechanisms behind this intricate system can empower you to navigate the financial landscape with greater awareness and control.
"Credit scoring models are a powerful tool that can be used to make informed lending decisions. However, it is important to remember that they are just a tool, and they should not be used as the sole basis for making credit decisions." - **Fair Isaac Corporation**
The world of credit scoring is a dynamic one, evolving with the rise of new data sources and advanced technologies. It's a realm where traditional models, long considered the gold standard, are now being challenged by sophisticated algorithms driven by machine learning.
But before we delve into the complexities of these modern models, it's crucial to understand the foundation upon which the credit scoring landscape is built. Let's embark on a journey to demystify the different types of credit scoring models and the factors they consider.
Traditional Credit Scoring Models: The Bedrock of Financial Assessment
For decades, the realm of credit scoring has been dominated by a handful of models, often referred to as traditional credit scoring models. These stalwarts, developed and refined over years of experience, have served as the bedrock of financial assessment. They are the cornerstone upon which lenders have made informed decisions, determining who gets credit and at what cost.
The most prominent among these traditional models is the FICO score, developed by the Fair Isaac Corporation (FICO). FICO scores, ranging from 300 to 850, are the gold standard in the United States, utilized by a vast majority of lenders to assess credit risk. These scores are meticulously calculated based on a five-factor system, each contributing to the overall score in a specific proportion:
Payment History (35%): This factor reflects your track record of paying bills on time, including credit card payments, loan payments, and utility bills. It's the most influential factor, indicating your reliability in fulfilling financial commitments. Late payments, missed payments, and defaults significantly impact this component, signaling a higher risk to lenders.
Amounts Owed (30%): This factor examines how much credit you're currently using compared to your total available credit. It reflects your level of indebtedness and your ability to manage existing financial obligations. A high credit utilization ratio, indicating a significant portion of your available credit being used, could signal a higher risk to lenders.
Length of Credit History (15%): This factor measures the duration of your credit history, the longer the better. A longer credit history demonstrates your experience in managing credit responsibly over an extended period. It signals stability and financial maturity to lenders, reinforcing your trustworthiness.
Credit Mix (10%): This factor considers the different types of credit you have, such as credit cards, mortgages, auto loans, and personal loans. A diverse mix of credit, demonstrating your ability to manage different types of financial products responsibly, is generally viewed favourably by lenders.
New Credit (10%): This factor assesses your recent credit activity, including new credit inquiries and newly opened accounts. Frequent credit applications can lower your score, signalling a potential increase in risk. A sudden surge in new credit lines can raise concerns about your ability to manage additional debt.
Another prominent traditional model is the VantageScore, developed through a collaboration of the three major credit bureaus: Equifax, Experian, and TransUnion. It uses a slightly different methodology than FICO, focusing on providing more consistent results across different credit reporting agencies. Like FICO, the VantageScore also ranges from 300 to 850, but its specific calculations may differ.
These traditional models have served as the backbone of the credit scoring system, providing lenders with a standardized approach to assessing creditworthiness. While they have proven effective, the increasing availability of data and advancements in technology have paved the way for a new era in credit scoring.
Behavioral Scoring Models: The Dynamics of Credit Behavior
While traditional models focus on a snapshot of your credit history, behavioural scoring models take a dynamic approach, analyzing your spending patterns, payment behaviour, and account usage over time. They aim to go beyond historical data, providing a more nuanced understanding of your creditworthiness by tracking your financial behaviour in real-time.
Imagine a credit card company analyzing your spending habits. They notice you consistently pay your bills on time, always stay within your credit limit, and rarely carry a balance. These positive behaviours could lead to a favourable score, potentially resulting in higher credit limits or lower interest rates.
Conversely, if you frequently make late payments, max out your credit cards, or engage in risky financial activities, your behavioural score might suffer, leading to higher interest rates or restricted credit access.
These models are often employed by banks and financial institutions to monitor existing customers' credit risk, helping them predict future behaviour and make more informed decisions about credit limits, interest rates, and even personalized offers.
Logistic Regression Models: Predicting Probabilities of Default
Imagine a scientist attempting to predict the likelihood of a plant blooming based on factors like sunlight exposure, water availability, and soil quality. Similarly, logistic regression models, a mainstay in credit scoring, seek to predict the probability of a borrower defaulting on a loan, considering various borrower attributes.
These models utilize statistical techniques to estimate the probability of default based on factors like income, debt levels, credit history, and employment status. This statistical approach provides lenders with a quantitative framework for evaluating credit risk, enabling them to make more informed lending decisions.
For instance, a lender using a logistic regression model might find that borrowers with a high debt-to-income ratio (DTI) have a higher probability of defaulting. This insight helps them adjust interest rates or credit limits for borrowers with a higher DTI, ensuring their portfolio remains balanced.
Machine Learning Models: The Power of Artificial Intelligence
In recent years, the realm of credit scoring has witnessed a paradigm shift, marked by the emergence of machine learning models. These advanced algorithms, powered by artificial intelligence, are revolutionizing how creditworthiness is assessed, offering unprecedented levels of accuracy and sophistication.
Imagine a chess grandmaster, meticulously analyzing the board, considering multiple moves simultaneously to outmaneuver their opponent. Machine learning models, similar to a chess grandmaster, can process vast amounts of data, uncovering complex, non-linear relationships that traditional models might miss. They can identify intricate patterns in borrower behaviour, economic indicators, and even social media data, providing a more comprehensive and dynamic view of credit risk.
These models, employing techniques like decision trees, random forests, gradient boosting, and neural networks, can capture nuanced details and adapt to changing circumstances, constantly refining their predictions.
Here are some key advantages of using machine learning in credit scoring:
Enhanced Accuracy: Machine learning algorithms can identify subtle patterns and relationships in data, leading to more accurate predictions of creditworthiness compared to traditional models.
Improved Risk Management: By accurately identifying high-risk borrowers, lenders can better manage their loan portfolios, minimizing potential losses.
Expanded Access to Credit: These models can help lenders extend credit to individuals with limited credit history, promoting financial inclusion.
Personalized Credit Offers: Machine learning enables lenders to personalize credit offers based on individual risk profiles, tailoring interest rates and credit limits to match individual needs.
However, the use of machine learning in credit scoring is not without its challenges. The complexity of these models, coupled with their ability to process vast amounts of data, can raise concerns about transparency and accountability. Regulators are actively scrutinizing these models to ensure they are fair, and unbiased, and do not perpetuate existing inequalities.
Custom Credit Scoring Models
Large financial institutions often operate in specialized niches, catering to particular customer segments with unique risk profiles. To effectively manage risk in these diverse environments, they often develop proprietary credit scoring models, tailored to their specific customer base and risk management preferences.
Imagine a lender specializing in mortgage loans, where factors like property value, income stability, and local housing market trends play a crucial role in assessing risk. Their custom credit scoring model would incorporate these specific factors, alongside traditional credit indicators, to provide a comprehensive assessment of a borrower's ability to repay a mortgage.
These custom models can include additional variables, such as social data, transaction patterns, and other non-traditional data sources. They can leverage advanced algorithms, drawing insights from alternative data sets to provide a more nuanced understanding of creditworthiness.
For example, a fintech company offering microloans might use a custom model that incorporates social media data, geolocation data, and mobile phone usage patterns to assess the creditworthiness of individuals with limited traditional credit history.
Economic-Based Credit Scoring: Accounting for Broader Economic Trends
Credit scoring is not a static concept. The financial landscape is constantly evolving, influenced by macroeconomic factors such as interest rates, inflation, and unemployment rates. These broader economic trends can significantly impact a borrower's ability to repay their debts.
Economic-based credit scoring models incorporate macroeconomic data into their calculations, adjusting credit scores based on the prevailing economic conditions. They aim to anticipate how external factors might impact a borrower's financial capacity and adjust their risk assessments accordingly.
Imagine a mortgage lender operating in an environment where interest rates are rising, potentially straining borrowers' ability to make monthly payments. An economic-based model would factor in these rising interest rates, adjusting the credit scores of borrowers to reflect the increased risk associated with these changing economic conditions.
These models are particularly relevant for lenders who are highly sensitive to market and economic changes, such as mortgage lenders, who often hold long-term loans with significant exposure to economic volatility.
Key Factors Shaping Your Credit Score: A Comprehensive Overview
While the specific factors considered in credit scoring models can vary, several key elements consistently influence your score. Understanding these factors can help you make informed decisions to improve your creditworthiness:
Payment History: This is the most influential factor, reflecting your reliability in fulfilling financial commitments. Paying bills on time, including credit card payments, loan payments, and utility bills, is crucial for a strong credit score.
Credit Utilization: This factor assesses how much credit you're currently using compared to your total available credit. Keeping your credit utilization ratio low (ideally below 30%) is essential for maintaining a healthy score.
Length of Credit History: The longer your credit history, the better. A longer history indicates experience in managing credit responsibly, signalling stability and financial maturity to lenders.
Credit Mix: Having a diverse mix of credit accounts, such as credit cards, mortgages, and auto loans, demonstrates your ability to manage different types of financial products responsibly.
New Credit: Frequent credit applications or opening new accounts too quickly can lower your score, signalling a potential increase in risk.
Hard Inquiries: Credit inquiries made by potential lenders can slightly lower your score. These inquiries are often made when you apply for new credit, such as a loan or a credit card.
Public Records: This includes information on bankruptcies, foreclosures, tax liens, and other legal actions that could affect your creditworthiness.
Debt Collections: If you have outstanding debts that have been sent to collections, it can negatively impact your score.
By understanding these key factors, you can take proactive steps to manage your credit effectively and build a strong credit score.
The Future of Credit Scoring: A Glimpse into the Financial Horizon
As technology continues to evolve and data becomes more abundant, the landscape of credit scoring is poised for further transformation. The future holds exciting possibilities for more sophisticated, personalized, and inclusive credit scoring models.
Alternative Data Sources: Credit scoring models are increasingly incorporating alternative data sources, such as social media activity, online spending patterns, and mobile phone usage patterns, to provide a more holistic view of creditworthiness. These data sources can help expand credit access to individuals with limited credit history, fostering financial inclusion.
Artificial Intelligence and Machine Learning: Advanced AI and machine learning algorithms are driving innovation in credit scoring, enabling models to capture complex, non-linear relationships in data, providing more accurate and dynamic predictions of credit risk.
Increased Transparency and Explainability: The growing use of complex machine learning models in credit scoring has raised concerns about transparency and explainability. The future of credit scoring likely involves greater efforts to ensure that models are fair, unbiased, and understandable to both borrowers and lenders.
Personalized Credit Scoring: As models become more sophisticated, they will be able to tailor credit scores and offers based on individual risk profiles, providing personalized financial solutions that better meet individual needs.
Real-Time Credit Scoring: The future may see real-time credit scoring, where models continuously analyze borrower behaviour, providing dynamic updates to credit scores, and reflecting evolving creditworthiness. This could lead to more responsive and agile lending decisions.
While the future of credit scoring is evolving rapidly, one thing remains constant: understanding the forces shaping your financial future is essential. By staying informed about credit scoring models, managing your credit responsibly, and advocating for transparency and fairness in credit scoring, you can navigate the financial landscape with confidence and create a brighter financial future for yourself.
Take control of your financial future! Explore your credit score, understand the factors that influence it, and adopt healthy credit habits. Stay informed about the latest developments in credit scoring and advocate for responsible, transparent, and inclusive practices. Your financial well-being depends on it.
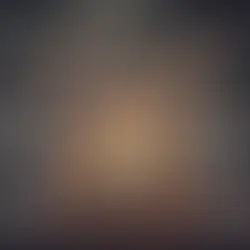
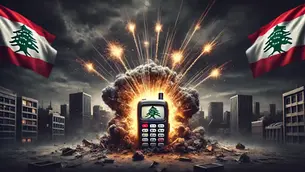
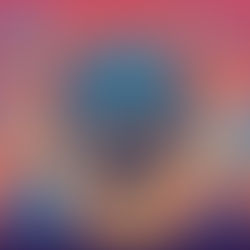
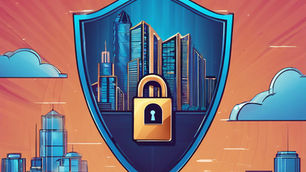
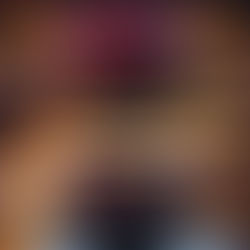
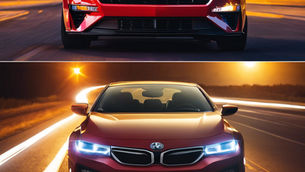
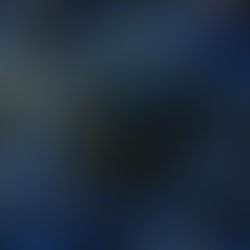
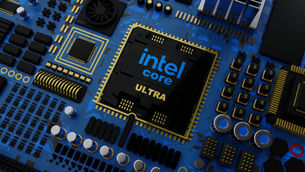