Probability of Default (PD)
Imagine you're a lender, ready to extend a helping hand to someone in need, offering them a loan to achieve their dreams. But, you're also a business, and responsible for your own financial well-being. How do you strike that balance between generosity and prudence? That's where the concept of Probability of Default (PD) comes in. It's like a compass guiding you through the murky waters of credit risk, helping you assess the likelihood of a borrower failing to repay their debt.
PD is not just a dry financial term; it's a vital gauge in the intricate dance of lending and borrowing. It's a measure that quantifies the risk of default, the dreaded moment when a borrower fails to meet their financial obligations. This risk, inherent in every loan, is the backbone of credit risk management, the art of navigating the unpredictable tides of debt repayment.
"The probability of default (PD) is a key input in credit risk modeling. It is the likelihood that a borrower will not be able to repay their debt on time." - **Credit Risk Management: A Practical Guide**
Delving Deeper into the Concept of PD
Defining the Unpredictable: Probability of Default
To understand PD, think of it as a number that represents the chances of a borrower defaulting on their loan within a specific time frame. The time frame is usually one year, but it can be adjusted depending on the type of loan and the requirements of the risk model.
Let’s say you’re a bank, and you’re considering lending money to a small business. The PD of that business is the chance that they won’t be able to pay back their loan within a year. The lower the PD, the less risk you face. The higher the PD, the more likely the borrower is to default, and the more risk you take on.
Beyond a Simple Number: Factors Influencing PD
Now, let’s dive a bit deeper. The PD of a borrower isn’t just a random number pulled out of a hat. It’s influenced by a whole host of factors, which can be broadly categorized into:
- Borrower-Specific Factors: These are the characteristics of the borrower themselves, which can affect their ability to repay their debt. These include:
- Macroeconomic Conditions: These are broader economic factors that can influence the overall economy and affect the likelihood of borrowers defaulting on their loans. These include:
- Industry-Specific Risks: These are risks that are specific to the industry in which a borrower operates. Some industries are naturally more volatile than others, and borrowers in these industries might have a higher PD. For example, borrowers in the energy sector might have a higher PD than borrowers in the healthcare sector, as the energy sector is more susceptible to fluctuations in commodity prices and government regulations.
Think of it like this: A small business owner operating in a stable industry with a strong track record of making payments, operating in a healthy economy, is less likely to default on a loan compared to a struggling business in a volatile industry, facing tough economic times.
The Impact of PD in Banking and Regulation
Navigating the Regulatory Landscape: Basel Framework
The Basel framework, a set of international regulations for banking supervision, recognizes the importance of PD in assessing credit risk. It requires banks to calculate PD, along with Loss Given Default (LGD) and Exposure at Default (EAD), to determine the amount of capital they need to hold against potential loan losses.
You can think of this as a bank's safety net for potential defaults. The Basel framework ensures banks have enough reserves to cushion the blow if borrowers start defaulting on their loans. This is crucial for maintaining financial stability in the banking sector.
Pricing Risk: How PD Influences Loan Interest Rates
PD plays a key role in setting interest rates on loans. A higher PD usually leads to a higher interest rate. Lenders charge higher interest rates to compensate for the increased risk of default. So, if a borrower has a higher PD, they'll typically pay a higher interest rate on their loan.
This is why credit scores are so important. If you have a good credit score, you're likely to get lower interest rates on loans, saving you money in the long run.
Stress Testing: Preparing for Turbulent Waters
Banks also use PD to perform stress tests, which simulate how their loan portfolios might perform under different economic scenarios. These tests help them assess how their loan losses might increase if the economy were to enter a recession or experience other adverse events.
Think of this as a “what-if” scenario. Banks want to know what might happen if the economy takes a downturn. This helps them prepare for potential losses and make adjustments to their lending practices accordingly.
Accounting for Losses: IFRS 9 and CECL
The IFRS 9 and CECL accounting standards, which are used by banks to account for their financial performance, also incorporate PD. These standards require banks to estimate expected credit losses, which take into account the probability of default.
This means banks are no longer just recognizing losses after they occur. They are now required to estimate potential losses based on PD and other factors, which helps them manage credit risk more proactively.
The Value of PD in Today's Financial Landscape
PD is an indispensable tool for managing credit risk. It helps lenders make informed decisions about who to lend to, how much to lend, and what interest rate to charge. This allows lenders to balance risk with profitability, ensuring they can continue to lend money while also mitigating the potential for losses.
As financial markets become more complex and interconnected, understanding and managing credit risk is more critical than ever. PD provides a powerful framework for doing just that. By using this tool effectively, lenders can help ensure a stable and sustainable financial system, enabling businesses and individuals to thrive.
Looking Ahead: The Future of PD
As technology continues to advance, we can expect to see even more sophisticated models and techniques for calculating PD. These models will likely incorporate a wider range of data sources, including alternative data such as social media activity and online behavior.
The use of artificial intelligence and machine learning is also likely to play a more prominent role in credit risk assessment, enabling lenders to develop more accurate and predictive PD models. This will help lenders to better understand and manage credit risk, leading to more efficient and effective lending decisions.
Conclusion
The world of finance is constantly evolving, and credit risk is a key challenge that lenders must address. Understanding and managing PD is crucial for ensuring the health and stability of the financial system. As we move into the future, we can expect to see even greater reliance on PD, helping lenders to make smarter and more responsible lending decisions.
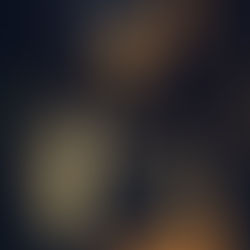

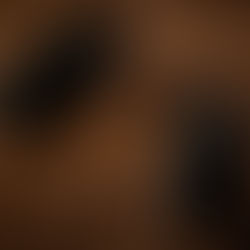
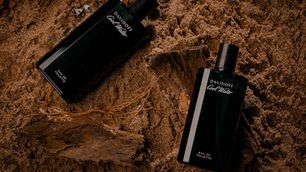
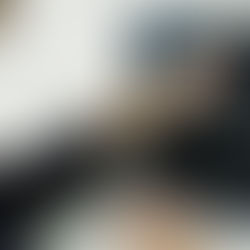
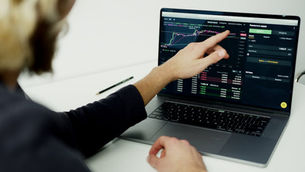

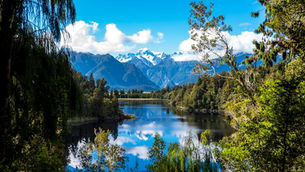